Understanding AI Traders: The Future of Investing
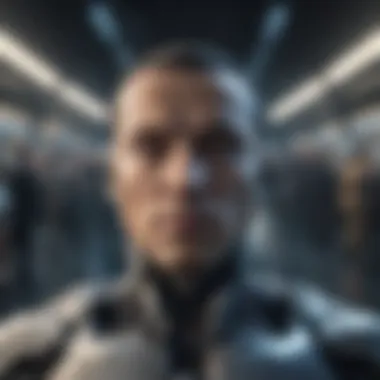
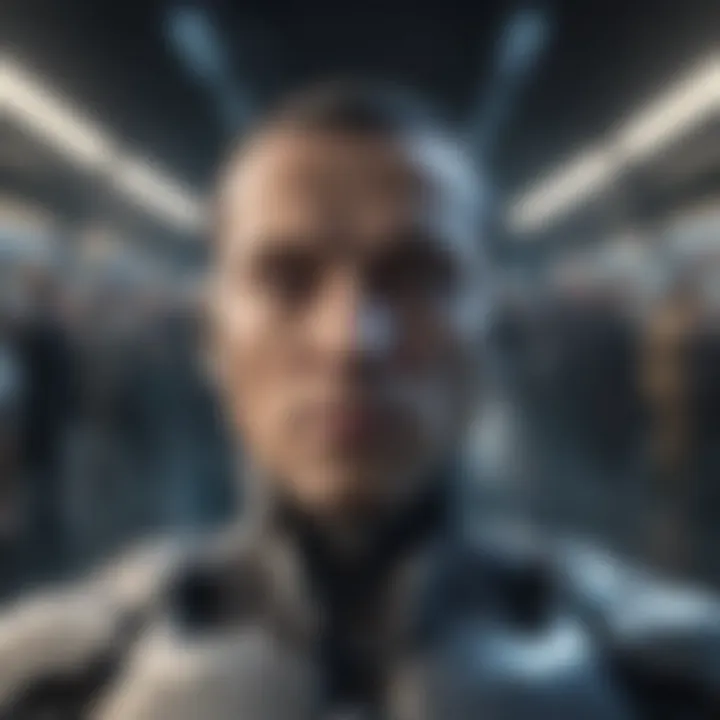
Intro
In recent years, the integration of artificial intelligence into financial trading has reshaped the investment landscape. This shift is not just about technology; it is fundamentally transforming how markets function. Understanding AI traders requires a grasp of the terms and concepts that define this domain.
AI traders utilize advanced algorithms and machine learning techniques to analyze market data, predict price movements, and execute trades. This evolution represents a culmination of years of technological advancement and changing market dynamics.
Investment Terminology
Definitions of Common Terms
Understanding the terminology is essential for navigating the world of AI trading. Here are some terms that appear frequently in discussions about AI traders:
- Algorithm: A set of rules or instructions designed to perform a task. In trading, algorithms analyze data and make decisions on buying or selling assets.
- Machine Learning: A subset of artificial intelligence that enables systems to learn from data, improving performance over time without explicit programming.
- High-Frequency Trading (HFT): A type of trading characterized by the use of algorithms to execute a large number of orders at extremely high speeds.
These terms form the foundation of the concepts discussed later in the article.
Industry Acronyms and Abbreviations
Familiarity with industry jargon can enhance comprehension. Here are some key acronyms:
- AI: Artificial Intelligence
- HFT: High-Frequency Trading
- ETF: Exchange-Traded Fund
- ROI: Return on Investment
These abbreviations frequently surface in conversations about trading technology and investment strategies.
Expert Insights and Advice
Strategic Investment Approaches
Investors interested in AI trading must consider various strategic approaches. Some notable strategies include:
- Trend Following: Algorithms analyze historical data to identify trends and make decisions to capitalize on these movements.
- Market Making: AI traders set buy and sell prices to facilitate liquidity in the market, profiting from the spread.
- Arbitrage: Utilizing price differences across different markets to secure a profit.
Risk Management Techniques
Effective risk management is vital for successful trading. Key techniques include:
- Stop-Loss Orders: Setting predetermined exit points to limit losses if a trade moves against an investor's position.
- Diversification: Reducing risk by spreading investments across various assets rather than concentrating them in one area.
- Stress Testing: Assessing how trading strategies will perform under different market conditions.
By incorporating these insights and strategies, investors can navigate the complexities of AI trading. This article aims to provide a comprehensive understanding of the intricacies involved in this rapidly evolving field, as well as the implications for the future of financial investments.
Preface to AI Trading
AI trading has emerged as a dominant force in the financial sector, reshaping how investments are made and managed. Understanding this phenomenon is crucial for investors at all levels. The integration of artificial intelligence into trading represents a paradigm shift that improves accuracy, speed, and efficiency in decision-making processes. Through algorithms that can analyze vast datasets, AI trading systems provide insights that are both deeper and more nuanced than traditional methods allow.
The importance of this topic in the broader context of financial investment cannot be overstated. With markets becoming increasingly complex, the ability of AI to process information in real time presents significant advantages. It transforms not just how trades are executed, but also how strategies are formulated and risks are managed.
By delving into AI trading, this article aims to reveal the underlying technology, advantages, challenges, and ethical considerations associated with this trend. The exploration begins with a clear definition of AI traders, followed by historical context—the foundations that have led us to this modern state of financial technology.
Definition of AI Traders
AI traders can be defined as computer programs that utilize artificial intelligence algorithms to analyze market data and execute trades. These systems leverage machine learning, neural networks, and other advanced computational techniques to predict market movements and optimize trading strategies. The essence of AI trading lies in its ability to process extensive datasets at speeds unattainable by human traders.
These tools can assess factors such as historical price trends, trading volumes, and even social media sentiment to anticipate future market behavior. The application of AI traders spans various areas including high-frequency trading, algorithmic trading, and robo-advisory services, creating a dynamic and competitive investment environment.
Historical Context
The roots of AI trading can be traced back to the late 20th century when quantitative analysis started to gain prominence. Early adopters utilized mathematical models to make investment decisions, but these methods were limited by computational power and data availability. As technology advanced, notably with the advent of the internet, access to real-time data became widespread.
In the early 2000s, with the development of machine learning techniques, the concept of AI trading began to take shape. Investors started to explore not just statistical models but also machine learning algorithms capable of learning from the data, refining their predictions over time. This era marked the transition from human-driven decision-making to technology-focused strategies.
The financial crisis of 2008 served as a catalyst for innovation in this field. As traditional methods faltered under the pressures of market volatility, AI trading systems gained traction. Today, AI is at the forefront of financial trading, significantly influencing how markets operate and how investors approach trading strategies.
Overall, understanding AI traders is essential for anyone involved in financial investment. It offers insights into a rapidly changing landscape and highlights the importance of technology in shaping investment outcomes.
Technological Foundations
In the realm of AI trading, understanding its technological foundations is essential. This section outlines the core components that make AI traders effective and efficient in today's fast-paced financial markets. With innovations rapidly emerging, the interplay between machine learning algorithms, data analysis capabilities, and real-time market data integration forms the backbone of artificial intelligence in finance. Together, these elements reshape trading strategies and define the future of investment.
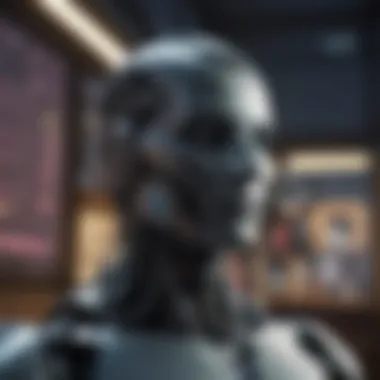
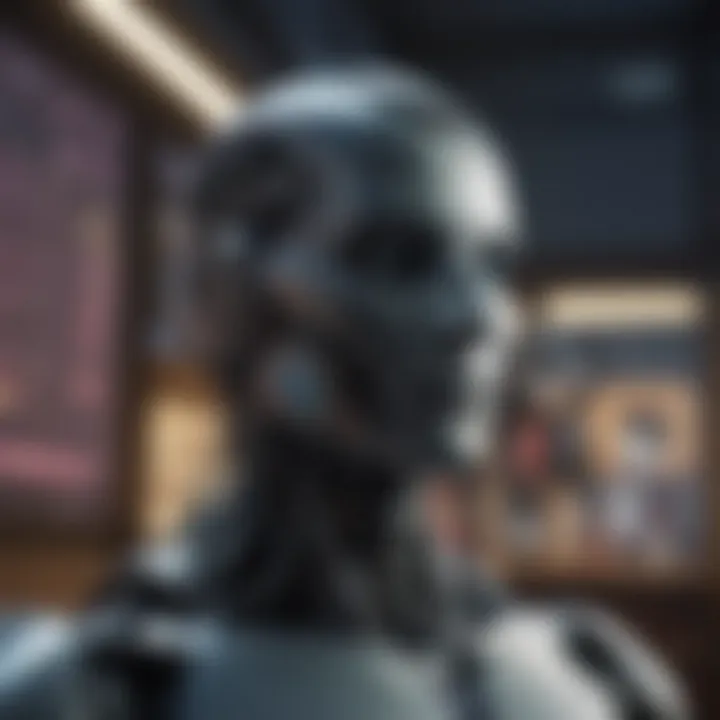
Machine Learning Algorithms
Machine learning algorithms serve as the heart of AI trading. They are designed to learn patterns in vast amounts of data, continually improving their accuracy over time. Traders can employ various algorithms, such as regression analysis, decision trees, and neural networks. Each type has its strengths and is suited for different trading objectives.
For instance, neural networks can analyze complex relationships in data, making them formidable for predicting price movements. By effectively processing historical data, these algorithms can generate trading signals that guide investment strategies. Not only do they improve decision-making precision, but they also adapt to changing market conditions, which is crucial in volatile environments.
Data Analysis and Processing
The importance of data analysis in AI trading cannot be overstated. High-quality data serves as a foundation for any successful trading strategy. AI systems analyze historical and real-time data to identify trends, anomalies, and potential opportunities.
Data processing involves cleaning, sorting, and organizing data from various sources, including market feeds, financial reports, and social media sentiment.
Key considerations include:
- Reliability of data sources
- Timeliness of data collection
- Volume of data handled
AI traders require sophisticated tools to manage and analyze immense datasets. Inadequate data can lead to misguided trading decisions, emphasizing the need for meticulous data processing.
Real-Time Market Data Integration
Real-time market data integration is another critical aspect of AI trading technologies. Financial markets operate round the clock, and the ability to process real-time data allows traders to react quickly to market changes. AI systems aggregate information from multiple sources, delivering insights that support rapid, informed decisions.
The benefits of real-time market data integration include:
- Improved responsiveness to news and events
- Enhanced forecasting of market movements
- Ability to optimize trading positions in real time
"Incorporating real-time data into AI trading systems is paramount for maintaining a competitive edge."
Effective integration ensures AI traders capitalize on fleeting opportunities, reducing the window for potential losses. As the financial landscape evolves, these technological foundations continue to be fundamental drivers of AI trading success, enabling both novice and seasoned investors to navigate complexities with greater confidence.
Advantages of AI Trading
AI trading represents a paradigm shift in how financial transactions are conducted in today's world. The increased use of artificial intelligence systems brings significant advantages that can improve trading outcomes, optimize asset management, and enhance operational efficiencies. These benefits are crucial for investors looking to navigate the complex and fast-paced financial markets effectively.
Enhanced Decision Making
One of the primary advantages of AI trading is the capability to enhance decision making. AI traders utilize complex algorithms to analyze vast amounts of market data. This process allows them to identify trends and patterns that may be difficult for human traders to discern.
AI systems can make decisions based on real-time data, quickly reacting to market changes. For instance, when specific market signals are detected—such as price fluctuations or significant shifts in trading volume—the AI can execute trades in fractions of a second. This speed and accuracy can lead to better trading outcomes compared to traditional methods.
Moreover, these algorithms can learn from historical data, improving their decision-making processes over time. By leveraging machine learning, AI traders refine their strategies based on past market behaviors, ultimately leading to more informed decisions.
Increased Efficiency
AI trading also delivers a remarkable increase in efficiency. In finance, time is a critical factor; the faster one can respond to market changes, the better the potential for profit. AI systems streamline numerous repetitive tasks that human traders ordinarily perform, such as data entry and analysis. This allows financial professionals to focus on more strategic aspects of investing.
With AI, data collection and processing are automated. It reduces the time taken to analyze trends and generate reports. An AI trader can monitor multiple markets simultaneously, which would be impossible for a human without significant resources. This capability leads to higher productivity and the possibility of managing larger portfolios.
Additionally, automation minimizes human error in trading practices. Algorithms function according to predefined parameters and can help eliminate emotional biases that often cloud human judgment.
Risk Management Capabilities
Risk management is another critical advantage of AI trading. AI systems are designed to assess risks more comprehensively. They can evaluate large datasets to identify potential risk factors and warn traders before making decisions that could lead to significant losses.
AI can simulate various market scenarios, predicting how certain conditions might affect asset prices. This predictive power enables traders to adjust their strategies accordingly, potentially avoiding downturns or seizing opportunities. For example, AI models can recognize when a market is becoming overly volatile, allowing traders to reposition their investments wisely.
Furthermore, AI's ability to respond to market data in real time means that risk management strategies can be applied immediately as the market changes. This adaptability is essential in high-stakes environments where timing can make or break investment success.
In summary, the advantages of AI trading include enhanced decision making, increased efficiency, and superior risk management capabilities. Understanding these benefits is vital for investors aiming to leverage technology in today's complex financial landscape.
Challenges and Limitations
As the integration of artificial intelligence in trading systems becomes more prevalent, understanding the inherent challenges and limitations is crucial. These aspects shape the effectiveness of AI traders in financial markets. Identifying these challenges helps investors make informed decisions while leveraging AI's benefits.
Market Volatility and Uncertainty
Market volatility represents the quantifiable changes in asset prices over time. AI traders depend heavily on historical data to identify trends and patterns. However, in highly volatile markets, such as those seen during economic downturns or crises, the data may not accurately predict future movements. This can lead to significant deviations from expected performance.
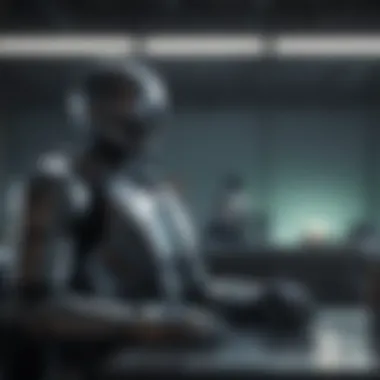
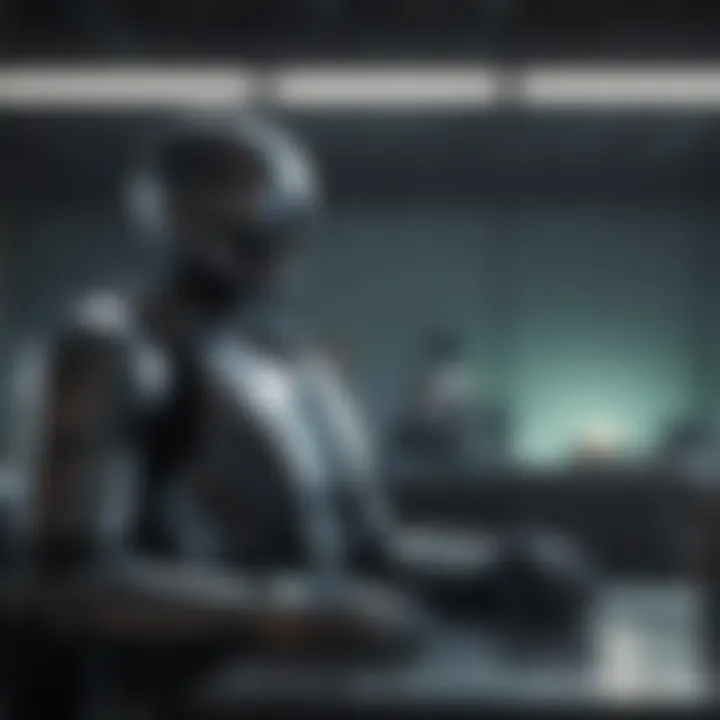
Uncertainty in the market can arise from various factors, including geopolitical events, economic shifts, and sudden market news. AI systems may lack the agility to adapt to unpredicted changes swiftly. As a result, traders may find themselves relying on outdated models that fail to account for rapid transformations, causing potential investment losses.
"Understanding market volatility is essential for effective AI trading strategies. Investors should not overlook the unpredictable nature of financial markets."
Dependence on Data Quality
AI traders are only as good as the data they use. High-quality data is critical for accurate predictions and decision-making. However, not all data sources are reliable. Poor data can lead to incorrect trend analysis and flawed trading signals. Additionally, the prevalence of biased information can skew an AI's learning process, resulting in systemic inaccuracies.
Moreover, ensuring data integrity is a continuous challenge. Data can become corrupted, outdated, or incomplete, thereby impacting the trading outcome. If investors do not prioritize data quality checks and validation, they risk the significant downside of algorithmic trading.
Potential for Algorithmic Errors
Algorithmic errors represent a considerable risk in AI trading. Even sophisticated algorithms can malfunction when faced with unusual market conditions or unforeseen inputs. An error in the code can trigger unintended trades, leading to financial losses. Because many AI trading systems operate on algorithms with minimal human oversight, the potential for cascading errors increases.
Furthermore, as AI systems evolve, they may develop complex behaviors that even their creators may not fully understand. This lack of transparency raises concerns about accountability. If an AI system makes a significant error, determining liability can become complicated. Investors must recognize these risks and have contingency plans in place when engaging with AI-driven trading strategies.
Ethical Implications
AI trading is not just about algorithms and financial gains; it also raises significant ethical questions that warrant careful consideration. When technology influences financial markets, the implications can extend beyond mere investment strategies to affect trust, employment, and regulation. Investors, regulators, and the wider society must grapple with these issues as they shape the future of financial investment. Understanding the ethical implications is crucial for ensuring that AI trading contributes positively to the economy and society as a whole.
Transparency in AI Decision Making
Transparency is a cornerstone of ethical AI trading. Investors and regulators must understand how decisions are made by AI systems. Algorithms often operate as black boxes, making it difficult to trace the path from data input to output decisions. This lack of clarity can erode trust among investors. When automated systems execute trades, it is essential to know whether their operations align with fair trading practices.
A transparent AI decision-making process can enhance accountability. If an algorithm creates a significant market shift or engages in questionable trading practices, stakeholders should be able to trace the decision's origins. Transparency helps to safeguard against manipulative strategies that could harm markets or individual investors.
To establish transparency, companies might need to adopt standards that outline how AI decisions are made. This might include documentation of the algorithms, data sources, and decision logic. Publicly available information about the AI systems used could foster confidence in their deployment while also facilitating regulatory oversight.
Implications for Employment
AI traders also present complex issues related to employment within the financial sector. As firms increasingly turn to automated systems, the traditional roles of traders, analysts, and other professionals may evolve or diminish. AI's ability to analyze vast datasets and execute trades at lightning speed might lead to significant changes in the labor market.
While automation can increase efficiency, it also poses the risk of job displacement. Workers must adapt to new tools and roles as the industry progresses. However, this does not mean all jobs will vanish. New roles may emerge within AI management, oversight, and development, requiring different skill sets. Educating current and future employees about AI systems is essential to ensure they remain relevant in a changing landscape.
Furthermore, companies should consider implementing strategies for workforce transition, such as retraining programs, to mitigate the risks associated with job loss. Emphasizing continuous learning can empower employees to adapt alongside technological advancements.
"The goal should not be to eliminate jobs but to transform the nature of work to make it more valuable for both people and organizations."
The ethical implications of AI trading extend beyond technology. They encompass a wide range of topics, including market fairness, trust, and the future of employment in the finance sector. Addressing these challenges is necessary for fostering a responsible approach to AI in financial investment.
Regulatory Considerations
Regulatory considerations in AI trading are crucial for maintaining market integrity and investor protection. As AI traders increasingly influence market dynamics, regulators must adapt to these changes. The landscape of financial regulation is evolving to address the unique challenges posed by automated trading systems. Understanding these regulations is essential for both seasoned investors and newcomers, as compliance impacts operational strategies and investment decisions.
Current Regulations Governing AI Trading
Currently, various regulatory bodies oversee AI trading practices. In the United States, the Securities and Exchange Commission (SEC) and the Commodity Futures Trading Commission (CFTC) enforce regulations that ensure fair trading practices. Some key regulations include:
- The Securities Exchange Act of 1934: This act mandates transparency and requires companies to disclose trading algorithms associated with high-frequency trading.
- Dodd-Frank Act: Enacted post-2008 financial crisis, it includes provisions aimed at increasing oversight of trading practices, particularly in derivatives markets.
- MiFID II (Markets in Financial Instruments Directive): In Europe, this legislation enhances transparency and improves investor protection, impacting AI trading as firms must report detailed transaction data.
These regulations help mitigate risks associated with algorithmic trading, including market manipulation and systemic risks. While many current regulations focus on traditional trading activities, they are starting to encompass AI-driven systems.
Future Regulatory Landscape
As technology continues to evolve, the future regulatory landscape will likely become more intricate. Possible developments might include:
- Tailored Regulations for AI Systems: Regulators may introduce specific guidelines for AI trading, including criteria for validating algorithms and managing non-compliance efficiently.
- Focus on Ethical AI Use: With increasing scrutiny on ethical considerations, future regulations may require transparency in algorithmic decision-making processes.
- Cross-Border Regulatory Collaborations: Given the global nature of financial markets, international cooperation among regulatory bodies will be necessary to ensure consistency in AI trading regulations.
"As AI technology matures, regulatory frameworks must adapt to ensure market fairness and protect investors from potential abuse."
In summary, adapting to the ever-changing landscape of AI trading regulations is essential for investors. Maintaining compliance not only safeguards the integrity of trading practices but also fosters investor confidence in the evolving financial ecosystem.
AI Trading Strategies
AI trading strategies are essential aspects of utilizing artificial intelligence in financial markets. These strategies leverage advanced technology to analyze vast data sets, identify patterns, and execute trades based on quantitative models. The importance of these strategies lies in their ability to outperform traditional trading methods, driven by speed, accuracy, and data processing capabilities.
Quantitative Trading
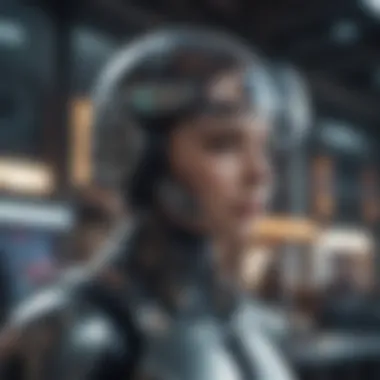
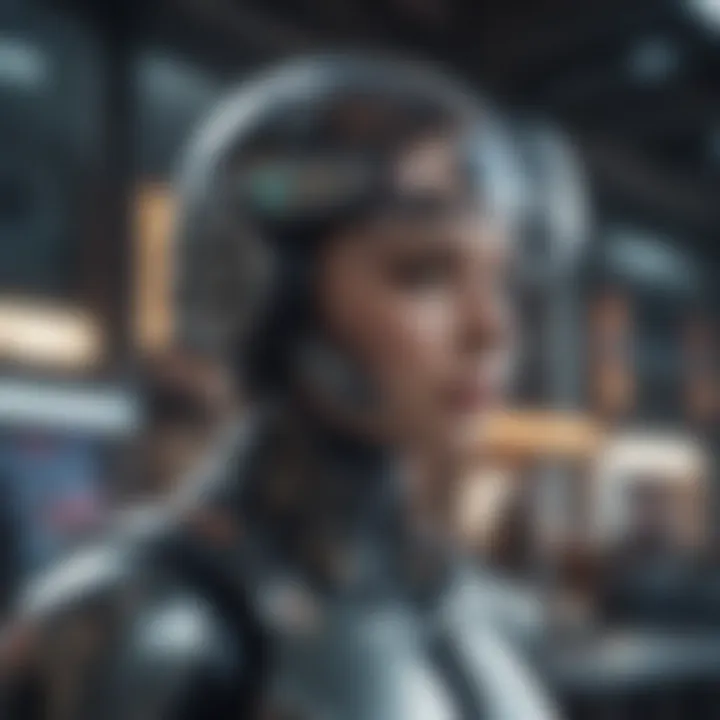
Quantitative trading refers to the use of mathematical and statistical models to predict market movements. This approach relies heavily on historical data, allowing traders to derive insights that may not be visible through conventional analysis.
Key features of quantitative trading include:
- Model-Driven Decisions: Trades are executed based on signals generated by algorithms evaluating market data. This reduces human bias and emotional influences.
- High-Frequency Trading: AI can process and react to market changes in milliseconds, allowing traders to capitalize on fleeting opportunities.
- Backtesting: Traders can evaluate their strategies against historical data to determine their effectiveness before deploying them in live markets.
Despite its many advantages, quantitative trading also poses challenges. For instance, market conditions may change rapidly, rendering previously successful models ineffective. Additionally, reliance on data quality is crucial, as inaccurate information can lead to poor trading decisions.
"Quantitative trading reshapes the landscape of investing, making data the cornerstone of decision-making."
Algorithmic Trading Models
Algorithmic trading models form the backbone of AI trading strategies. These models automate the process of executing orders based on predetermined criteria. They can analyze multiple instruments and markets, allowing traders to diversify their portfolios efficiently.
Some important aspects of algorithmic trading models include:
- Execution Speed: Algorithms can execute trades much faster than human traders, capturing price movements more effectively.
- Precision: Algorithms can minimize slippage and transaction costs by placing orders at optimal moments, enhancing profitability.
- Flexibility: Traders can customize algorithms for different market conditions, adjusting parameters based on volatility or liquidity.
However, the reliance on algorithms introduces risks as well. Technical glitches can lead to erroneous trading decisions, and external factors such as regulatory changes can impact their effectiveness. It is crucial for investors to continuously monitor and adjust their algorithms to maintain competitive advantages in the market.
The Role of AI in Personal Investment
The integration of artificial intelligence into personal investment strategies has become increasingly significant as technology evolves. AI in personal investment not only enhances the efficiency of asset management but also improves decision-making quality. Understanding the implications and applications of AI in this domain provides valuable insights for both beginner and seasoned investors.
Wealth Management Applications
Wealth management is a crucial aspect of personal finances, and AI offers innovative methods to optimize this process. AI-driven platforms analyze vast datasets to identify trends and correlations that might not be apparent to human analysts.
- Personalized Investment Strategies: AI can create customized investment plans based on individual risk tolerance, financial goals, and market conditions. This personalization ensures that investment strategies align closely with each investor's needs.
- Performance Monitoring: Many wealth management tools utilize AI to continuously monitor investment performance. This real-time analysis allows investors to make informed adjustments quickly to enhance returns.
- Predictive Analytics: By employing advanced algorithms, AI predicts market movements and asset performance, aiding investors in making proactive rather than reactive decisions. This capability can lead to better outcomes in various market scenarios.
Robo-Advisors and Hybrid Models
Robo-advisors represent an innovative approach to wealth management powered by AI. These platforms automate investment strategies, often at a lower cost than traditional financial advisors. They appeal to a broad audience and democratize access to investment services.
- Cost-Effective Services: Robo-advisors typically charge lower fees compared to human advisors, making investment more accessible to novice investors. This affordability often includes portfolio management services that were once reserved for wealthier clients.
- Ease of Use: These platforms provide user-friendly interfaces, ensuring that even those with limited financial knowledge can navigate the investment process without difficulty.
- Hybrid Models: Some firms combine traditional financial advisory services with robo-advice, creating hybrid models. These offer personalized touchpoints while leveraging AI’s efficiency in data analysis. This model helps bridge the gap between automated systems and personal advisory, appealing to diverse investor preferences.
The role of AI in personal investment is transforming how individuals approach financial growth, making strategies more efficient and tailored to personal needs.
In summary, AI's impact on personal investment is profound. Wealth management applications, along with the evolution of robo-advisors and hybrid models, signify a shift toward more personalized, efficient, and accessible investment solutions. Investors must stay informed about these developments to maximize their financial strategies.
Future Trends in AI Trading
The evolution of AI technology in trading is still in full swing. Emerging trends signal changes that could redefine the landscape of financial investments. These changes include advancements in Natural Language Processing and the integration of AI with Blockchain technology. Understanding these trends is crucial for investors seeking to stay ahead in the competitive markets.
Advancements in Natural Language Processing
Natural Language Processing, or NLP, is a remarkable field of AI that enables machines to understand human language. Recent advancements in NLP allow AI traders to analyze news articles, social media, and even earnings reports with greater accuracy.
- Sentiment Analysis: One application allows traders to gauge market sentiment. By analyzing text data, AI can assess whether the overall sentiment is positive, negative, or neutral, enabling better investment decisions.
- Automated Reporting: AI can also generate reports based on the data it analyzes. This can save time and provide insights that are more current than traditional methods.
- Real-Time Insights: With NLP, AI systems can produce real-time analyses of ecomomic events, which is crucial for swift market reactions.
In summary, advancements in NLP empower AI traders to process large volumes of information quickly. This capability is vital for making informed decisions in a fast-paced financial environment.
Integration of AI with Blockchain Technology
The integration of AI with Blockchain technology is a developing trend that can enhance transparency and security in trading. Blockchain provides a decentralized and immutable ledger, while AI enables data analysis and predictive modeling. The combination opens numerous possibilities:
- Enhanced Security: AI algorithms can detect anomalies and fraudulent activities on Blockchains. This can provide an added layer of security in trading transactions.
- Smart Contracts: AI can improve the functionality of smart contracts by making them more adaptive and capable of handling complex conditionality. This can streamline trading processes and reduce errors.
- Data Analytics: Blockchain generates vast amounts of data. With AI, traders can harness this data to predict market trends or optimize trading strategies more effectively.
The future of AI trading is closely tied to these emerging technological trends. As investors adapt and embrace these innovations, the financial investment landscape continues to evolve.
Finale
The conclusion of this article serves to synthesize the essence of AI trading and its significance in the financial investment landscape. Understanding AI traders is not merely an academic exercise; it is crucial for grasping how modern investment strategies are evolving. As we've seen throughout the sections, the intersection of advanced technologies and finance has its profound implications.
Summary of Findings
The exploration encompassed various dimensions of AI trading, providing a comprehensive overview. Here are the key points:
- Technological Advancements: The core of AI trading lies in machine learning algorithms and data analytics, which enable traders to process large volumes of market data substantially faster than traditional methods.
- Advantages: Increased efficiency, enhanced decision-making, and stronger risk management capabilities stand out. These advantages allow investors to navigate complex market conditions more adeptly.
- Challenges: The elements of market volatility, dependency on data quality, and the potential for algorithmic errors cannot be ignored. They require continuous reassessment and adaptation of strategies to mitigate risks.
- Ethical and Regulatory Aspects: The ethical implications of AI in trading, particularly regarding transparency and employment impacts, must inform future practices. Regulatory measures will need to evolve to catch up with rapid technological changes.
- Future Trends: Advancements in natural language processing and blockchain technology integration will likely shape the future landscape of AI trading, pushing the boundaries of traditional finance further.
Final Thoughts on AI Trading
In closing, AI trading represents a major shift in how investors approach the market. The future landscape will undoubtedly feature a more prominent role of technology in everyday trading decisions. While there is undeniable potential for improved outcomes, it is imperative for investors to remain wary of the accompanying challenges and ethical questions. By being informed and engaged, both novice and seasoned investors can navigate this evolving field more effectively.
Understanding the implications of AI in finance is not just beneficial for strategic advantage; it is essential for participation in the modern market. As technology continues to advance, the integration of AI into investment strategies will redefine financial frontiers. The road ahead may be uncertain, but the importance of adapting to it is clear.